By Lom Seunbane, Chief Strategy Officer
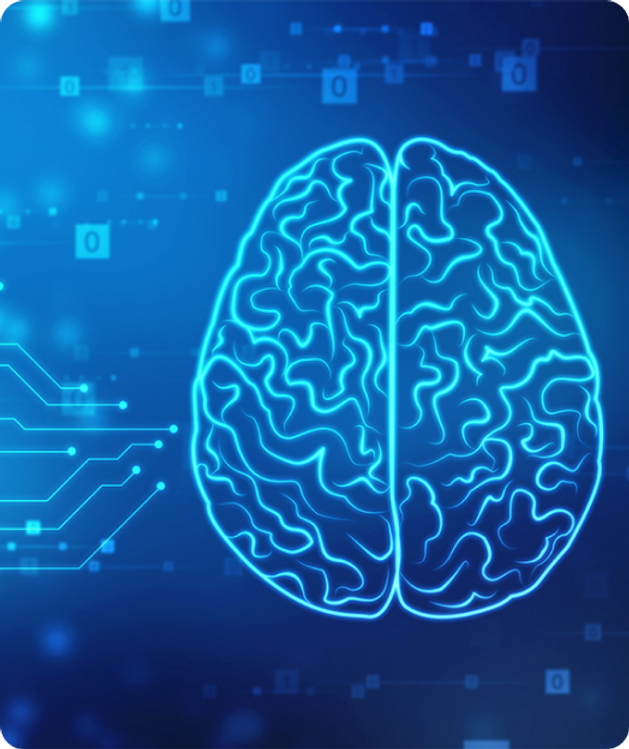
Machine Learning (ML) has been an important aspect of many business solutions since the turn of the millennium. Put simply, ML is about computer algorithms that can improve itself or perform tasks without being explicitly programmed to do so. It can do this through “experience,” which is data it gains through some medium or by its original “training,” which is data you feed the algorithm in the beginning. You probably noticed that the key in both scenario is data.
DATA IS KING!
What has made ML so effective as a business solution/maker in the past two decades has been the rate at which we can collect data. It’s the reason Facebook and Google has been so successful, whether for better or worse. Every webpage you visit, every item you purchase at the supermarket, or even what your face looks like as you drive is collected as data. It’s a bit scary when you think about it, but it has also led to some amazing applications. For instance, speech recognition wouldn’t have been possible without the data collected on how we speak. Because of that, accessibility on everything from phones to laptops has been improved.
It’s not a surprise to hear that data is important for creating business strategies. A compelling use for data collection is understanding customer behavior. Items purchased, browsing time, or even cards used for payment can help tell a story. And through ML, we don’t even need to know how these attributes relate to one another.
MACHINES CAN THINK BETTER THAN US
ML grew out of our quest for artificial intelligence. We believed that machines can learn from data and thus, learned to think for themselves. However, AI of the period gravitated towards high-level symbolic AI, which was a bit different from the probabilistic models of ML. This caused ML to reorganize into a separate field and it started to flourish in the 1990s. Instead of trying to achieve artificial intelligence, the goal of ML changed itself to focus on tackling solvable problems of a practical nature, incorporating much of statistics and probability theory.
Today, we use a lot of ML, together with data mining, to make predictions, whether it is quantitative or qualitative. In making our predictions, we take different “features” (in math terms, the independent variables) and we run them through different ML modeling approaches with the end result a predictive model (comparable to the dependent variable). In a standard two-variable system (having only one X), this would be the “line of best fit.” However, in most ML problems, we usually have many features so our problem would have many X variable input and thus, be multidimensional. All of this is to say why ML is so amazing. It can take all these features and visualize how they are all connected when even the human mind cannot comprehend that many dimensions.
A good example to illustrate machine learning is predicting crop yield. We know that irrigation affects crops. So does sunlight, and perhaps even wind and soil PH. But we don’t know how much each feature (to use ML parlance) affects the crop output. Data scientists can take these four features and set them as X1, X2, X3, and X4. We run the data through a modeling approach we choose (which itself can be a complicated process) and in the end, we will have a model. The modeling result will also tell us the accuracy of the model. If all the features had an impact on crop yield, we would end up with a high accuracy model. Then, we can get current information on those features we used and input them into the model and predict what the crop yield would be in the future.
CONCLUSION
It should be no wonder to you how such a predictive tool can be powerful in business. It is why we at Magic Box Solutions experiment quite a bit with ML to see how we can best use it to serve our clients. We cannot discuss it openly at the moment, but one such project of ours focus on using machine learning for sound and image recognition. It’s an exciting time, and one in which we believe will revolutionize how we conduct business.
By Lom Seunbane, Chief Strategy Officer
Machine Learning (ML) has been an important aspect of many business solutions since the turn of the millennium. Put simply, ML is about computer algorithms that can improve itself or perform tasks without being explicitly programmed to do so. It can do this through “experience,” which is data it gains through some medium or by its original “training,” which is data you feed the algorithm in the beginning. You probably noticed that the key in both scenario is data.
DATA IS KING!
What has made ML so effective as a business solution/maker in the past two decades has been the rate at which we can collect data. It’s the reason Facebook and Google has been so successful, whether for better or worse. Every webpage you visit, every item you purchase at the supermarket, or even what your face looks like as you drive is collected as data. It’s a bit scary when you think about it, but it has also led to some amazing applications. For instance, speech recognition wouldn’t have been possible without the data collected on how we speak. Because of that, accessibility on everything from phones to laptops has been improved.
It’s not a surprise to hear that data is important for creating business strategies. A compelling use for data collection is understanding customer behavior. Items purchased, browsing time, or even cards used for payment can help tell a story. And through ML, we don’t even need to know how these attributes relate to one another.
MACHINES CAN THINK BETTER THAN US
ML grew out of our quest for artificial intelligence. We believed that machines can learn from data and thus, learned to think for themselves. However, AI of the period gravitated towards high-level symbolic AI, which was a bit different from the probabilistic models of ML. This caused ML to reorganize into a separate field and it started to flourish in the 1990s. Instead of trying to achieve artificial intelligence, the goal of ML changed itself to focus on tackling solvable problems of a practical nature, incorporating much of statistics and probability theory.
Today, we use a lot of ML, together with data mining, to make predictions, whether it is quantitative or qualitative. In making our predictions, we take different “features” (in math terms, the independent variables) and we run them through different ML modeling approaches with the end result a predictive model (comparable to the dependent variable). In a standard two-variable system (having only one X), this would be the “line of best fit.” However, in most ML problems, we usually have many features so our problem would have many X variable input and thus, be multidimensional. All of this is to say why ML is so amazing. It can take all these features and visualize how they are all connected when even the human mind cannot comprehend that many dimensions.
A good example to illustrate machine learning is predicting crop yield. We know that irrigation affects crops. So does sunlight, and perhaps even wind and soil PH. But we don’t know how much each feature (to use ML parlance) affects the crop output. Data scientists can take these four features and set them as X1, X2, X3, and X4. We run the data through a modeling approach we choose (which itself can be a complicated process) and in the end, we will have a model. The modeling result will also tell us the accuracy of the model. If all the features had an impact on crop yield, we would end up with a high accuracy model. Then, we can get current information on those features we used and input them into the model and predict what the crop yield would be in the future.
CONCLUSION
It should be no wonder to you how such a predictive tool can be powerful in business. It is why we at Magic Box Solutions experiment quite a bit with ML to see how we can best use it to serve our clients. We cannot discuss it openly at the moment, but one such project of ours focus on using machine learning for sound and image recognition. It’s an exciting time, and one in which we believe will revolutionize how we conduct business.